Project Members
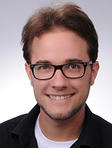
Christian Wiwie
(Former group member)
ClustEval is a free and extendable opensource platform for objective performance comparison of arbitrary Clustering Methods on different datasets. It is designed to support the standard processes related to Cluster Analyses.
Thanks to highly advanced wet-lab techniques, we are produceing a tremndous amount of biological data everyday. This wealth of data urges for sophisticated automatic knowledge extraction techniques. One of the most popular techniques is clustering, i.e., the grouping of similar objects into clusters.
Even though clustering is a long standing problem in computer science, conducting a high-quality cluster analysis is all but straight forward. For the practitioner, the very plethora of existing clustering algorithms is already a huge obstacle. Each tool requires at least one parameter and does not perform equally well on every dataset. Finding the optimal tool and paramter setting is a very tiresome and error-prone process.
With ClustEval, we introduce an integrated clustering framework, assisting the user in all steps of cluster analyses, from data preprocessing and parameter optimization to evaluating the reported clusters. The framework allows the fully automated execution of many different tool on given datasets and an exhaustive evaluation. The flexibility of the framework allows convenient extension with new tools, datasets, and quality measures. Furthermore, the website summarizes the results of millions of cluster evaluations providing an excellent overview of the current state-of-the-art clustering tools.
Christian Wiwie
(Former group member)