Projects
MOPITAS
Multi-OMICS Profiling in Time and Sapce
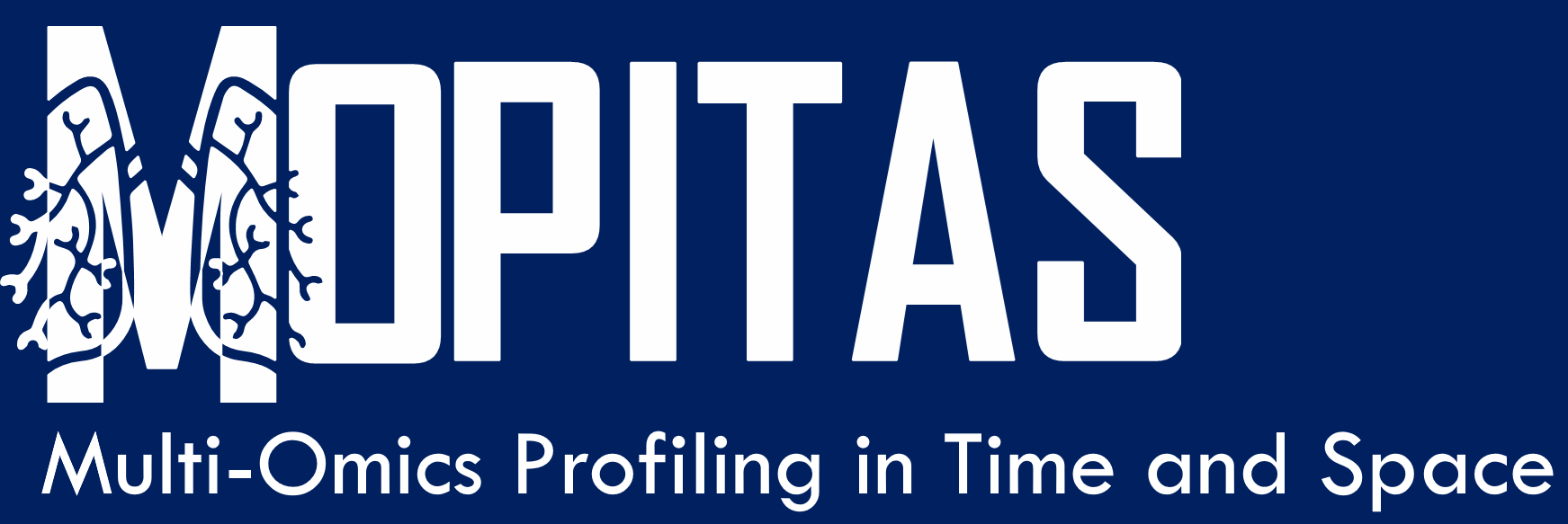
The role of cells in the tissue is largely defined by their differential gene expression,
which unfolds both in space and in time. Recent advances in Spatial Transcriptomics
(ST) allow for assaying gene expression in tissues, however current workflows offer
limited spatial resolution or do not yield genome-wide expression levels. Conversely,
single-cell RNA sequencing (scRNA-seq) is a mature, cost-effective technology that
captures more RNA transcripts and identifies individual cells adequately. With this
transformative project, we seek to bridge the gap between ST and scRNA-seq by
developing innovative data science solutions and deep neural networks that are
capable of identifying the spatial relationship of the sequenced cell based on an ST
map. In contrast to existing methods, which seek to complete the low transcriptomic
resolution of ST by enriching it with a likely expression profile from the connected single
cell experiment, we will actually place the single cells in their correct location in space,
taking into account the neighborhood of the single cells in their tissue. This tremendous
leap forward in accuracy and the account for sensitive neighborhood relationships
coupled with novel integrative approach, we will be able to unravel spatially resolved
genetic programs across different tissues, organisms, and developmental stages, in
particular at the interface between different cell types in complex tissues. Furthermore,
current methods are only working with well-categorized, stereotypical tissues like the
brain but fail with complex tissues. Here, our innovative co-creation-based wet-lab
approach will produce data targeted to feed the data science pipelines by sampling
tissues of increasing complexity allowing transfer learning from simpler to complex
tissues. Our algorithms will pave the way to truly single-cell resolved spatial
transcriptomics for complex tissues.
read more
Screen4Care
Shortening the path to rare disease diagnosis by using newborn genetic screening and digital technologies

A new international consortium aims to tackle the major hurdle for rare disease patients – the lengthy and convoluted
diagnosis journey – via an innovative research approach based on two central pillars: genetic newborn screening and
artificial intelligence (AI)-based tools for the early identification of undiagnosed patients.
The project will run for a period of five years with a total budget of EUR 25 million provided by the Innovative
Medicines Initiative, a joint undertaking of the European Union and the European Federation of Pharmaceutical
Industries and Associations (EFPIA).
SDU will contribute in particular with our expertise in federated machine learning and the establishment of a novel
federated metadata repository amenable to machine learning. Here, the sensitive data never leaves the save harbor of
storage, but nevertheless, complex machine models can be trained. Ultimately, rare disease patients shall be quicker
identified by training artificial intelligence on medical records and thus shorten the journey of these patients to
diagnosis and treatment.
Further Information can be found on the project website: https://screen4care.eu
read more
Publications
(Raycheva et al., 2024)
(Garnier et al., 2023)
-
Ralitsa Raycheva, Kostadin Kostadinov, Elena Mitova, Georgi Iskrov, Georgi Stefanov, Merja Vakevainen, Kaisa Elomaa, Yuen-Sum Man, Edith Gross, Jana Zschüntzsch and others. Landscape analysis of available European data sources amenable for machine learning and recommendations on usability for rare diseases screening. Orphanet Journal of Rare Diseases 19(1): 1–18 (2024).
-
Nicolas Garnier, Joanne Berghout, Aldona Zygmunt, Deependra Singh, Kui A Huang, Waltraud Kantz, Carl Rudolf Blankart, Sandra Gillner, Jiawei Zhao, Richard Röttger and others. Genetic newborn screening and digital technologies: A project protocol based on a dual approach to shorten the rare diseases diagnostic path in Europe. Plos one 18(11): e0293503 (2023).
Villum Experiemnt MS2AI
Artificial Intelligence to revolutionize Protein Mass Spectrometry
Protein mass spectrometry is the major technique to characterize thousands of proteins in
biological samples, and therefore is an important cornerstone for unraveling and understanding
biological processes and their role in complex diseases. Although this experimental platform is
increasingly used in biological and clinical research, the current instrumentation is very
expensive, slow and requires experienced technical staff for their operation. One reason for this
complexity is the usage of at least two stages of data acquisition to ensure high quality
identification and quantification of proteins.
We seek to revolutionize this slow and costly process by stripping the second stage from the
experiment and replace it with cutting-edge artificial intelligence algorithms, so-called deep
learning models. These AIs will be trained to predict proteins on basis of extensive mostly
unharvested information from the first stage. We envision that our method will open protein
mass spectrometry to a broader spectrum of use-cases in research and industry, and ultimately
to enable high-quality research with small mobile units.
read more
Publications
(Rehfeldt et al., 2023)
(Rehfeldt et al., 2023)
(Rehfeldt et al., 2022)
(Rehfeldt et al., 2021)
-
Tobias Greisager Rehfeldt, Konrad Krawczyk, Simon Gregersen Echers, Paolo Marcatili, Pawel Palczynski, Richard Röttger and Veit Schwämmle. Variability analysis of LC-MS experimental factors and their impact on machine learning. GigaScience 12: giad096 (2023).
-
Tobias Greisager Rehfeldt, Konrad Krawczyk, Simon Gregersen Echers, Paolo Marcatili, Pawel Palczynski, Richard Röttger and Veit Schwaemmle. Variance Analysis of LC-MS Experimental Factors and Their Impact on Machine Learning. bioRxiv: 2023–05 (2023). Link.
-
Tobias Greisager Rehfeldt, Konrad Krawczyk, Mathias Bøgebjerg, Veit Schwämmle and Richard Röttger. MS2AI: Automated repurposing of public peptide LC-MS data for machine learning applications. Bioinformatics 38(3): 875–877 (2022). Link.
-
Tobias Greisager Rehfeldt, Konrad Krawczyk, Mathias Bøgebjerg, Veit Schwämmle and Richard Röttger. MS2AI: Automated repurposing of public peptide LC-MS data for machine learning applications. bioRxiv (2021). Link.
AIERE
Machine learning-based solutions for automatic image enhancement in real estate
Currently, image enhancements in the real estate market are tremendously labor-intensive to meet the
high quality-standards and are outsourced to low-income countries. Those tasks include the rather
simple enhancements like color and exposure correction but also range from changing the mood of
images to even replace furniture. Even though the images are manually processed, the quality of the
results show large variations and inconsistencies.
In this project, we aim to develop novel deep neural network tools to automatize several of the most re-occurring tasks
in professional image copy-editing. Here, only the highest quality of outputs are sufficient for the professional
setting and all images have to be processed in full camera resolution.
This is an industrial PhD project, conducted with our industry partner Esoft A/S.
read more
Publications
(missing reference)
(Marı́n-Vega Juan et al., 2022)
-
Juan Marı́n-Vega, Michael Sloth, Peter Schneider-Kamp and Richard Röttger. DRHDR: A dual branch residual network for multi-bracket high dynamic range imaging. In Proceedings of the IEEE/CVF Conference on Computer Vision and Pattern Recognition. (2022): 844–852. Link.
FeatureCloud
Privacy preserving federated machine learning and block chaining for reduced cyber risks in a world of distributed healthcare
The digital revolution, in particular big data and artificial intelligence (AI) offer new opportunities to transform
healthcare. However, it also harbors risks to the safety of sensitive clinical data stored in critical healthcare ICT
infrastructure. In particular, data exchange over the internet is perceived insurmountable, posing a roadblock hampering
big data based medical innovations.
With FeatureCloud, we are involved in a pan-European transformative AI development project, which implements a software
toolkit for substantially reducing cyber risks to healthcare infrastructure by employing the world-wide first
privacy-by-architecture approach, which has two key characteristics: (1) no sensitive data is sent through any
communication channels, and (2) data is not stored in one central point of attack.
We are in particular responsible for the creation of federated unsupervised methods, dealing with the privacy-aware
federated preprocessing and clustering.
More Information can be found on the project website: https://featurecloud.eu
read more
Publications
(Hartebrodt & Röttger, 2022)
(Hartebrodt et al., 2022)
(Hartebrodt & Röttger, 2022)
(Hartebrodt et al., 2021)
(Torkzadehmahani et al., 2020)
(Matschinske et al., 2021)
(Matschinske et al., 2021)
-
Anne Hartebrodt and Richard Röttger. Privacy of federated QR decomposition using additive secure multiparty computation. arXiv preprint arXiv:2210.06163 (2022). Link.
-
Anne Hartebrodt, Reza Nasirigerdeh, Jan Bamubach, David Benjamin Blumenthal, Tim Kacprowski and Richard Röttger. Medical data safety via federated machine learning. In RExPO22 Conference. (2022). Link.
-
Anne Hartebrodt and Richard Röttger. Federated Horizontally Partitioned Principal Component Analysis for Biomedical Applications. Bioinformatics Advances (2022). Link.
-
Anne Hartebrodt, Reza Nasirigerdeh, David B Blumenthal and Richard Röttger. Federated Principal Component Analysis for Genome-Wide Association Studies. In 2021 IEEE International Conference on Data Mining (ICDM). (2021): 1090–1095. Link.
-
Reihaneh Torkzadehmahani, Reza Nasirigerdeh, David B Blumenthal, Tim Kacprowski, Markus List, Julian Matschinske, Julian Späth, Nina Kerstin Wenke, Béla Bihari, Tobias Frisch, Anne Hartebrodt, Anne-Christin Hausschild, Dominik Heider, Andreas Holzinger, Walter Hötzendorfer, Markus Kastelitz, Rudolf Mayer, Cristian Nogales, Anastasia Pustozerova, Richard Röttger, Harald H.H.W. Schmidt, Ameli Schwalber, Christof Tschohl, Andrea Wohner and Jan Baumbach. Privacy-preserving Artificial Intelligence Techniques in Biomedicine. arXiv preprint arXiv:2007.11621 (2020). Link.
-
Julian Matschinske, Julian Späth, Reza Nasirigerdeh, Reihaneh Torkzadehmahani, Anne Hartebrodt, Balázs Orbán, Sándor Fejér, Olga Zolotareva, Mohammad Bakhtiari, Béla Bihari, Marcus Bloice, Nina C Donner, Walid Fdhila, Tobias Frisch, Anne-Christin Hauschild, Dominik Heider, Andreas Holzinger, Walter Hötzendorfer, Jan Hospes, Tim Kacprowski, Markus Kastelitz, Markus List, Rudolf Mayer, Mónika Moga, Heimo Müller, Anastasia Pustozerova, Richard Röttger, Anna Saranti, Harald HHW Schmidt, Christof Tschohl, Nina K Wenke and Jan Baumbach. The FeatureCloud AI Store for Federated Learning in Biomedicine and Beyond. arXiv preprint arXiv:2105.05734 (2021). Link.
-
Julian Matschinske, Nicolas Alcaraz, Arriel Benis, Martin Golebiewski, Dominik G Grimm, Lukas Heumos, Tim Kacprowski, Olga Lazareva, Markus List, Zakaria Louadi, Josch K Pauling, Nico Pfeifer, Richard Röttger, Veit Schwämmle, Gregor Sturm, Alberto Traverso, Kristel Van Steen, Martiela Vaz Freitas, Gerda Cristal Villalba Silva, Leonard Wee, Nina K Wenke, Massimiliano Zanin, Olga Zolotareva, Jan Baumbach and David B Blumenthal. The AIMe registry for artificial intelligence in biomedical research. Nature methods 18(10): 1128–1131 (2021). Link.
Software
MS2AI
From Mass Spectrometry to Artificial Intelligence
MS2AI is the official pipeline to get Mass spectrometry data into machine learning applications.
The Pipeline does everything from data acquisition, data extraction and machine learning applications,
making it the one-stop software for MS and AI implementations.
MS2AI fully automates the retrieval and processing of MS projects deposited on Pride or in your local research
environment. The projects are retrieved, filtered and stored in a machine learning suitable format. We additionally
provide sample deep neural networks, which can be trained on the generated data extracts.
For documentation and source code, visit the git repository here
read more
Publications
(Rehfeldt et al., 2022)
(Rehfeldt et al., 2021)
-
Tobias Greisager Rehfeldt, Konrad Krawczyk, Mathias Bøgebjerg, Veit Schwämmle and Richard Röttger. MS2AI: Automated repurposing of public peptide LC-MS data for machine learning applications. Bioinformatics 38(3): 875–877 (2022). Link.
-
Tobias Greisager Rehfeldt, Konrad Krawczyk, Mathias Bøgebjerg, Veit Schwämmle and Richard Röttger. MS2AI: Automated repurposing of public peptide LC-MS data for machine learning applications. bioRxiv (2021). Link.
Scellnetor
Scellnetor is a novel clustering tool for scRNA-seq data that takes Scanpy generated AnnData objects in H5AD
file-format as input. With Scellnetor you can compare two sets of cells that you manually select on one of your
Scanpy-generated plots. The output will be connected components of genes where the genes are either differently or
similarly expressed in the two sets. You can also do a clustering of a single set, where the genes in the connected
components are similarly expressed. For every cluster, you get a plot showing mean gene expression and the genes’
95 % confidence intervals and a table with statistically significant GO-terms.
For full documentation and the webservice, visit the Scellnetor page here.
read more
Publications
(Grønning et al., 2020)
-
Alexander GB Grønning, Mhaned Oubounyt, Kristiyan Kanev, Jesper Lund, Tim Kacprowski, Dietmar Zehn, Richard Röttger and Jan Baumbach. Comparative single-cell trajectory network enrichment identifies pseudo-temporal systems biology patterns in hematopoiesis and CD8 T-cell development. bioRxiv (2020). Link.
TiCoNE
Time Course Network Enrichment
We developed time course network enrichment (TiCoNE), a novel human-augmented time series clustering method combined
with a temporal network enricher that enables drug target discovery based on temporal networks. Temporal gene clusters
are embedded into molecular networks, and TiCoNE identifies molecular pathways (subnetworks) with a differential
behavior under two conditions (e.g., diseases). Such temporal disease pathway candidates are evaluated by calculating
empirical p-values.
TiCoNE works with most kinds of biological entities (genes, proteins, RNAs, etc.) and most types of molecular measures
acquirable for them (transcriptomics,proteomics, etc.). It comes as web server and as Cytoscape plugin.
Web: https://ticone.compbio.sdu.dk/
read more
Publications
(Wiwie et al., 2017)
(Wiwie et al., 2019)
(Wiwie et al., 2019)
-
Christian Wiwie, Alexander Rauch, Anders Haakonsson, Inigo Barrio-Hernandez, Blagoy Blagoev, Susanne Mandrup, Richard Röttger and Jan Baumbach. Elucidation of time-dependent systems biology cell response patterns with time course network enrichment. arXiv preprint arXiv:1710.10262 (2017). Link.
-
Christian Wiwie, Irina Kuznetsova, Ahmed Mostafa, Alexander Rauch, Anders Haakonsson, Inigo Barrio-Hernandez, Blagoy Blagoev, Susanne Mandrup, Harald HHW Schmidt, Stephan Pleschka, Richard Röttger and Jan Baumbach. Time-Resolved Systems Medicine Reveals Viral Infection-Modulating Host Targets. Systems Medicine 2(1): 1–9 (2019). Link.
-
Christian Wiwie, Richard Röttger and Jan Baumbach. TiCoNE 2: A Composite Clustering Model for Robust Cluster Analyses on Noisy Data. arXiv preprint arXiv:1904.12353 (2019). Link.
ClustEval
A Clustering Evaluation Framework
ClustEval is a free and extendable opensource platform for objective performance comparison of arbitrary Clustering
Methods on different datasets. It is designed to support the standard processes related to Cluster Analyses.
Thanks to highly advanced wet-lab techniques, we are produceing a tremndous amount of biological data everyday. This
wealth of data urges for sophisticated automatic knowledge extraction techniques. One of the most popular techniques
is clustering, i.e., the grouping of similar objects into clusters.
Even though clustering is a long standing problem in computer science, conducting a high-quality cluster analysis is
all but straight forward. For the practitioner, the very plethora of existing clustering algorithms is already a huge
obstacle. Each tool requires at least one parameter and does not perform equally well on every dataset. Finding the
optimal tool and paramter setting is a very tiresome and error-prone process.
With ClustEval, we introduce an integrated clustering framework, assisting the user in all steps of cluster analyses,
from data preprocessing and parameter optimization to evaluating the reported clusters. The framework allows the fully
automated execution of many different tool on given datasets and an exhaustive evaluation. The flexibility of the
framework allows convenient extension with new tools, datasets, and quality measures. Furthermore, the website
summarizes the results of millions of cluster evaluations providing an excellent overview of the current
state-of-the-art clustering tools.
Web: http://clusteval.sdu.dk
read more
Publications
(Wiwie et al., 2015)
(Wiwie et al., 2018)
(Wiwie & Röttger, 2017)
-
Christian Wiwie, Jan Baumbach and Richard Röttger. Comparing the performance of biomedical clustering methods. Nature methods 12(11): 1033–1038 (2015). Link.
-
Christian Wiwie, Jan Baumbach and Richard Röttger. Guiding biomedical clustering with ClustEval. Nature protocols 13(6): 1429–1444 (2018). Link.
-
Christian Wiwie and Richard Röttger. On the Power and Limits of Sequence Similarity Based Clustering of Proteins Into Families. In Pacific Symposium on Biocomputing 2017. (2017): 39–50. Link.